How to effectively Optimise & Scale your business
- Marino Pernía G.
- Mar 2
- 5 min read
Updated: Mar 3
Why Optimisation Matters
Even the most sophisticated AI tools will degrade in value if they aren’t continuously refined. As your business processes evolve, so must your AI models, data pipelines, and automation workflows. Gartner estimates that nearly 85% of AI projects stall because organisations treat AI as a one-and-done solution rather than a system that requires ongoing upkeep. By proactively optimising your AI initiatives, you ensure they stay aligned with both current business goals and emerging market demands.
“Research from Forrester shows that 65% of AI adopters see diminishing returns after 12 months if they don’t perform ongoing model updates and process refinements.”
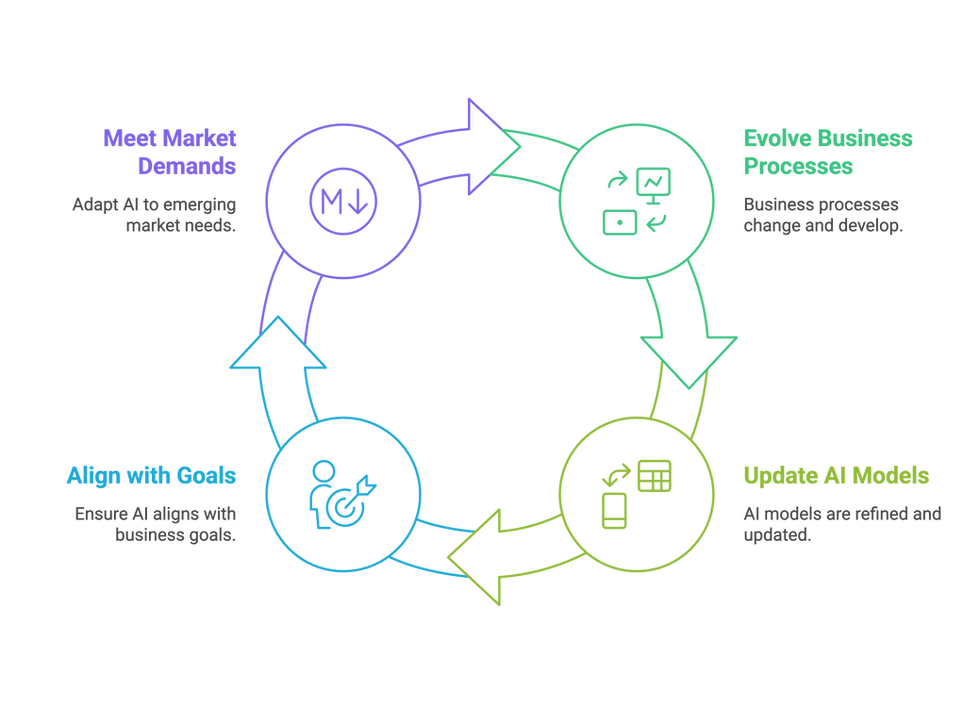
Fine-tune your models and processes
Continuous Model Improvement
For AI-driven lead generation or predictive analytics, periodic re-training of models is crucial. Over time, data drifts—customer behaviour changes, new competitors emerge, and your original dataset grows stale. Regularly re-training your models ensures you’re always working with up-to-date insights:
Schedule re-training: Depending on how fast your industry moves, aim for quarterly or semi-annual refreshes.
Monitor performance metrics: Keep an eye on accuracy, precision, and recall for predictive models, or on lead-to-conversion rates for lead-gen AI.
Add new data: If you’ve expanded into new products, regions, or demographics, fold that fresh data into your training sets.

Refining Workflows
Once your Intelligent Process Automation (IPA) or administrative automation is in place, process refinement can yield surprisingly large gains. Gather user feedback and track bottlenecks in your newly automated workflows. Minor adjustments—like reordering tasks or adjusting queue times—can free up hours per week.
Example: If your AI-based scheduling tool consistently overbooks prime time slots, tweak the system’s logic or capacity constraints. This small fix often drastically improves user experience without requiring an entirely new system.
“A B2B software company that implemented AI for lead scoring re-trained their model quarterly. As a result, their lead-to-opportunity rate improved from 28% to 35% within a year, simply by regularly updating the data sets.”

Measure Real Business Outcomes
During Stage 2, you likely tracked initial adoption and efficiency gains. Now, shift focus to long-term business impact. For each AI or automation project, define specific Key Performance Indicators (KPIs) that tie directly to revenue or cost savings. Examples include:
Lead Generation:
Increase in qualified leads by X%
Reduced acquisition cost by Y%
Operations:
Time saved per workflow cycle
Percentage decrease in rework or errors
Customer Service:
Customer Satisfaction Score (CSAT) or Net Promoter Score (NPS) improvements
24/7 coverage ratio—i.e., how many queries are fully resolved by AI outside business hours
Automating data collection is essential for consistent reporting. Tools like Boomi’s process intelligence can integrate metrics from various systems (CRM, helpdesk, finance) into a single dashboard, making it easy to see big-picture improvements.
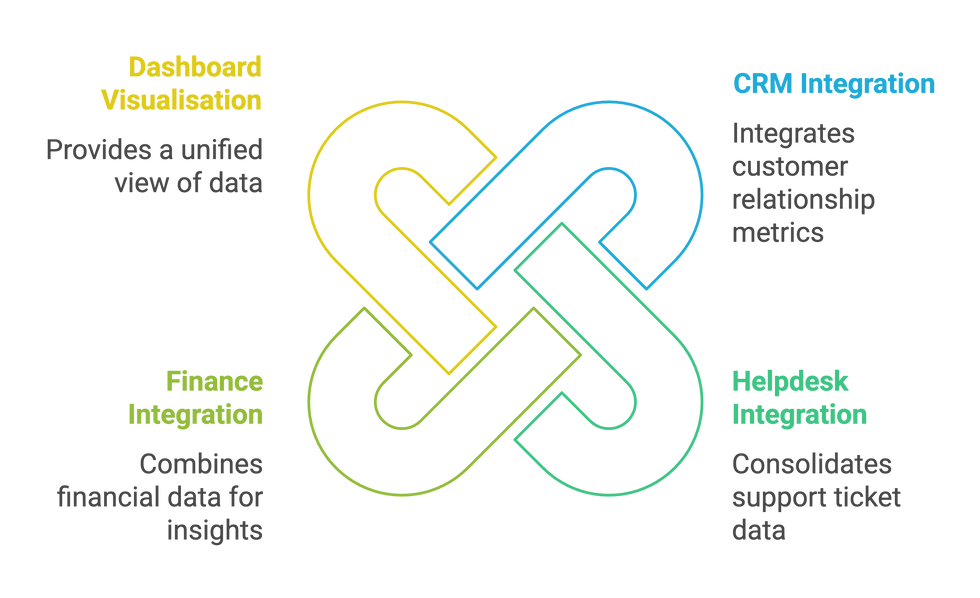
Scale AI Capabilities Across Teams
Internal Knowledge-Sharing
Once you’ve proven ROI in one department—say, using AI to handle 60% of customer service queries—scale that success to adjacent teams. Share best practices and success stories across the company:
Lunch-and-Learn Sessions: Host monthly internal workshops to demonstrate how AI is improving processes in each department.
AI Center of Excellence: Consider forming a small cross-functional team that oversees new AI rollouts and ensures consistent quality.
Industry-Specific Scaling Tips
Architecture & Design
After successfully using tools like ARCHITEChTURES for small-scale residential projects, consider extending AI to large commercial developments or even city planning. Work with your AI vendor or in-house data scientists to handle more complex variables (e.g., advanced zoning constraints or environmental impact) while maintaining design quality.
Real Estate
Real estate firms can expand from basic lead qualification to AI-driven property matchmaking. Once your AI has proven its ability to identify high-potential buyers, scale that same logic to different property categories—residential, commercial, luxury segments—and unify these data streams in a single CRM. This broadens your addressable market while maintaining a high standard of client personalisation.
Photographers & Artists
If you’ve used AI for automating client scheduling and payment, the next step might be AI-assisted editing or image tagging to manage large photo libraries. For artists, consider AI tools that analyze client preferences to propose new creative directions. Over time, you can even scale to an e-commerce platform that automatically curates galleries for individual visitors based on their browsing patterns.
Educators & Coaches
Educational institutions can move from basic administrative automation to advanced personalized learning paths. If your AI solution effectively identifies each student’s strengths and weaknesses, scale it to new programs or entire grade levels. Introduce collaborative AI tools for instructors to share lesson plans, success stories, and best practices in real time.
Build a Culture of Continuous Improvement
Iteration Over Perfection
The biggest threat to scaling AI is waiting for a perfect system that never arrives. Instead, aim for small, iterative updates:
Plan: Identify an improvement or new feature.
Build: Implement it in a controlled environment or pilot group.
Measure: Gather performance data.
Refine: Use feedback to fix bugs or tweak configurations.
Deploy: Roll it out widely.
Encourage Experimentation
Empower employees to suggest new use cases for AI and automation. Offer micro-grants or dedicated experimentation time so teams can prototype ideas. Some of your best scaling wins may come from an intern or a front-line employee who sees an efficiency gap no one else has noticed.
Pitfalls to avoid in the optimisation phase:
Complacency: Assuming your AI is ‘done’ and letting it run on autopilot.
Ignoring User Feedback: If employees find shortcuts or workarounds, it might indicate the AI or process isn’t meeting real needs.
Lack of Unified Metrics: Different departments tracking success differently can lead to conflicting priorities.
Future-Proof Your Tech Stack
As AI evolves, so should your infrastructure. Keep an eye on emerging technologies like generative AI for design or text creation, or reinforcement learning for complex decision-making. Evaluate whether your existing systems can accommodate these new approaches without a major overhaul.
Checklist for future-proofing:
Cloud-Native: Host your solutions on scalable platforms (AWS, Azure, Google Cloud).
API-First: Ensure easy integration with new AI modules or microservices.
Data Governance: Maintain robust privacy and compliance frameworks, especially if you’re collecting sensitive customer data.
Vendor Relationships: Regularly review your AI vendors’ roadmaps to see how their product updates align with your growth plans.
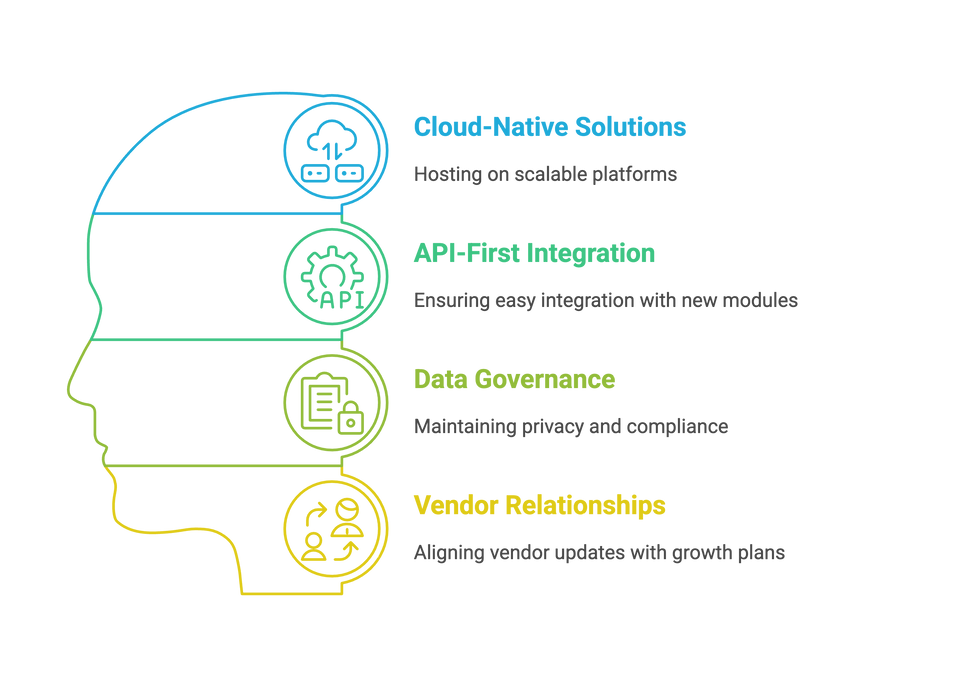
Wrapping Up the Growth Playbook
By the end of Stage 3, you’re not just “using AI”—you’re scaling it. You’ve built the processes, metrics, and culture to continually refine and expand your AI capabilities in tandem with your business objectives.
Key Takeaways:
Continuous Model Updates: AI thrives on fresh data and iterative re-training.
Real Business KPIs: Measure success by tangible ROI, not vanity metrics.
Phased Rollouts: Start small, prove the value, then expand across teams or verticals.
Culture of Improvement: Encourage experiments, celebrate incremental wins, and never let your AI stack go stagnant.
With these three stages—Discover & Strategise, Implement, and Optimise & Scale—you now have a holistic roadmap for weaving AI and automation into every corner of your business. Done right, the payoff is massive: streamlined operations, happier customers, and a robust competitive edge that grows stronger with every data point your AI collects.
Ready to fine-tune and scale your AI systems for maximum impact? Let’s talk about how we can help you level up.
Commentaires