How to effectively Implement AI & Automation in your business
- Marino Pernía G.
- Mar 2
- 5 min read
Updated: Mar 3
In our first post of this Growth Playbook series, we explored the crucial discovery phase – identifying where AI and automation can deliver the biggest impact for your business. Now it's time to roll up our sleeves and tackle the implementation phase – where good intentions meet practical execution.
“McKinsey reports that well-structured AI implementations can improve operational efficiency by 20–40%, but only if teams are trained adequately.”

Moving from strategy to action
Let's be honest – this is where most businesses stumble. They've got a shiny new strategy deck gathering digital dust while day-to-day operations continue unchanged. Implementation isn't just about buying software; it's about integrating these tools into your actual workflow without derailing productivity.
The businesses that pull ahead are those that approach implementation with both urgency and discipline. According to research, organisations employing predictive maintenance systems can anticipate equipment failures before they occur, creating efficiency gaps that non-adopters struggle to close. But this advantage only materialises when implementation is handled thoughtfully.
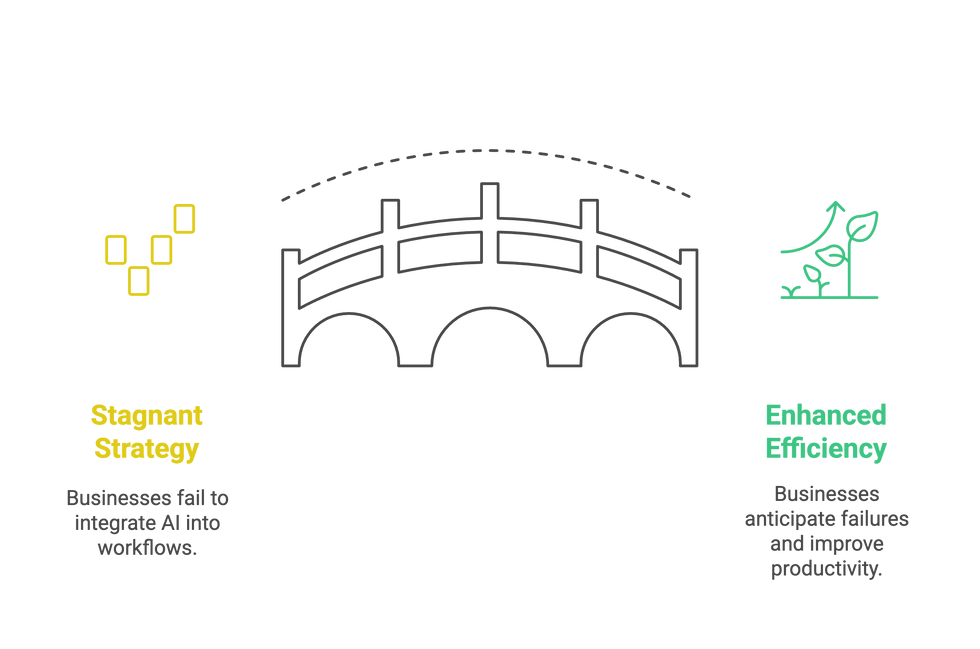
The four-step implementation framework
Our Playbook breaks implementation into four manageable phases designed to minimise disruption while maximising adoption:
1. Select the right tools for your specific needs
The AI marketplace is flooded with options – from specialised solutions to comprehensive platforms. Your selection criteria should include:
Integration capabilities: How easily will it connect with your existing systems?
Scalability: Can it grow with your business?
Training requirements: What's the learning curve for your team?
Support options: What happens when issues arise?
For lead generation specifically, look for systems that can rapidly optimise strategies by analysing interaction patterns, providing a multidimensional view of potential customers that enables precise targeting.
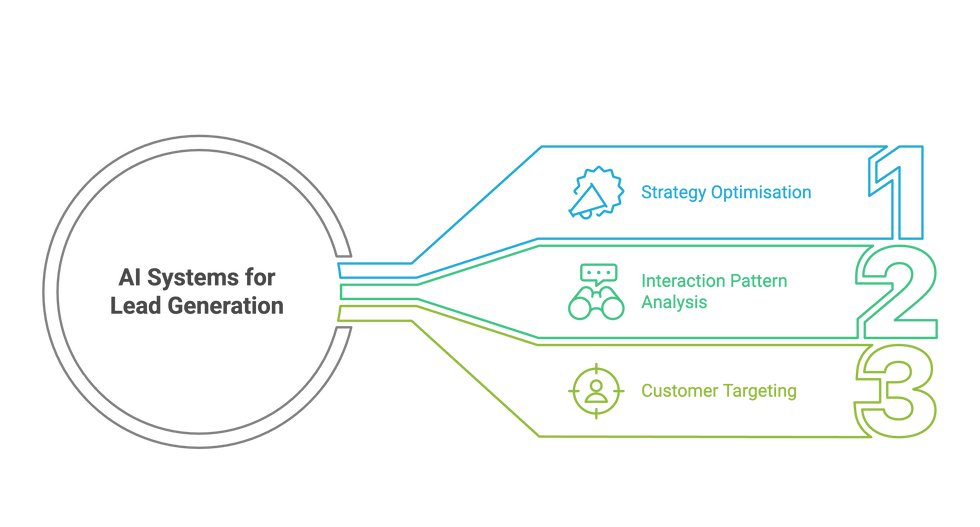
2. Pilot before full deployment
Resist the urge to go all-in immediately. Instead:
Choose a single department or process for your pilot
Set clear, measurable objectives for the pilot period
Collect structured feedback from all stakeholders
Be prepared to make adjustments before wider rollout
For customer service implementations, test your AI-powered virtual assistants with a subset of common queries before handling your entire support volume. This allows you to refine responses and identify edge cases before they impact customer satisfaction.
“For example, a healthcare startup piloted AI-driven appointment scheduling in just one department. Within six weeks, they saw a 25% drop in no-shows and a 10% boost in patient satisfaction scores.”
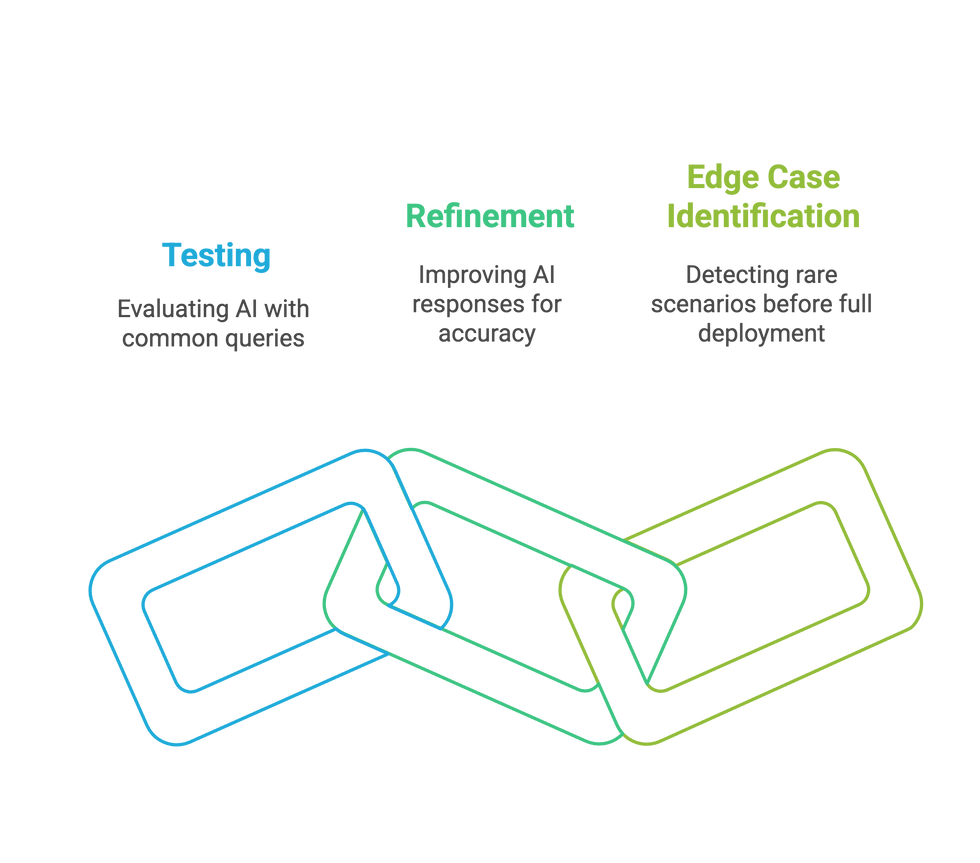
3. Invest in proper training and change management
New tools mean nothing without adoption. Your implementation plan must include:
Role-specific training: Different team members need different skills
Champions program: Identify and empower internal advocates
Process documentation: Clear guidelines for new workflows
Ongoing support: Resources for troubleshooting and optimisation
When implementing intelligent process automation for administrative functions, invest extra time training your administrators. They'll become your in-house experts and can help refine the system to better match your specific business needs.
Pitfalls to Watch Out For:
Skipping the pilot: Rolling out company-wide on day one can overwhelm staff and create chaos.
Poor change management: If your team doesn’t understand the ‘why’ behind the new tools, adoption rates plummet.
Inconsistent measurement: Without baseline metrics, you can’t gauge whether the new system is delivering ROI.

4. Measure, Adjust, and Iterate
Implementation isn't a one-and-done event but an ongoing process:
Establish baseline metrics before implementation
Track key performance indicators weekly
Schedule regular review sessions with stakeholders
Create a feedback loop for continuous improvement
Implementation Phase | Common Pitfalls | Success Strategies |
Selection | Feature overload, budget creep | Focus on core needs, start with essential features |
Piloting | Too narrow scope, insufficient testing | Include edge cases, test with real scenarios |
Training | Information overload, resistance to change | Chunked learning, clear "what's in it for me" |
Measurement | Vanity metrics, inconsistent tracking | Focus on business outcomes, automate data collection |

Industry-specific implementation insights
Each industry faces unique challenges during the implementation phase. Here's what to watch for:
Architecture & Design implementation
For architectural firms implementing tools like ARCHITEChTURES, the key challenge is balancing AI assistance with creative control. Start by using the AI for routine aspects of projects while maintaining human oversight on design decisions. Gradually increase AI involvement as confidence in the system grows.
Create implementation milestones that first focus on using AI for optimising basic residential layouts before moving to more complex commercial projects. This progressive approach builds both technical capability and team confidence.
Real Estate implementation
Real estate businesses should begin with implementing AI for lead qualification rather than completely automating client interactions. The human touch remains vital in property transactions, so design your implementation to enhance rather than replace personal service.
Your CRM should be the central hub of your AI implementation, with automation tools feeding qualified leads to agents based on property specialisation and past success rates. This hybrid approach maintains the relationship aspect of real estate while leveraging AI for efficiency.
Photographers & Artists implementation
Creative professionals should implement AI tools first for business administration rather than creative processes. Start with client management systems that automate scheduling, invoicing, and routine communications before expanding to creative assistance.
When implementing portfolio management systems, carefully tag and categorise past work to train the AI effectively. This creates a foundation for more sophisticated applications like style analysis and client preference matching later in your implementation journey.
Educators & Coaches implementation
For educational businesses, phased implementation is crucial. Begin with administrative automation to handle enrolment and scheduling, then gradually introduce personalised learning tools as both instructors and students grow comfortable with the technology.
The most successful implementations combine AI capabilities with human instruction, using technology to identify learning gaps that teachers then address personally. This complementary approach maintains educational quality while enhancing efficiency.
Overcoming Implementation Obstacles
Even the best-planned implementations face challenges. Here's how to navigate the most common ones:
Data Quality Issues
AI systems are only as good as the data they learn from. Before full implementation:
Audit your existing data for completeness and accuracy
Establish data cleaning protocols
Create governance standards for ongoing data collection
Integration Challenges
Legacy systems can create implementation headaches. Mitigate this by:
Mapping all data flows between systems before implementation
Using middleware solutions where direct integration isn't possible
Budgeting for potential custom development needs
Resistance to Change
Perhaps the biggest implementation challenge is human rather than technical. Address this by:
Involving end-users in the selection process
Clearly communicating how new tools will make their jobs easier
Celebrating early wins and sharing success stories
Providing above-and-beyond support during the transition period
The Implementation Timeline
While every business moves at its own pace, a typical implementation follows this timeline:
Weeks 1-2: Tool selection and procurement
Weeks 3-4: System configuration and integration
Weeks 5-6: Pilot program with limited scope
Weeks 7-8: Training and change management
Weeks 9-10: Controlled rollout to wider organisation
Weeks 11-12: Optimisation based on initial feedback
This compressed timeline works for smaller businesses implementing specific tools. Enterprise-wide implementations typically extend this timeline by 3-4x, with corresponding phases for each department or function.
Measuring Implementation Success
Implementation isn't complete until you've verified results. Track these metrics:
Adoption rate (% of team actively using the new tools)
Efficiency gains (time saved per process)
Error reduction (% decrease in mistakes or rework)
ROI (measurable financial returns compared to implementation costs)
The implementation phase sets the stage for our final Growth Playbook component: Optimise & Scale. A thoughtful implementation creates the foundation for continuous improvement and expansion of your AI capabilities.
In our next and final post, we'll explore how to take your successfully implemented AI systems to the next level, optimising performance and scaling capabilities across your entire organisation.
Want a customised implementation roadmap for your specific business? Let's connect.
Comments